An In-Depth Look at Quantitative Trading Strategies
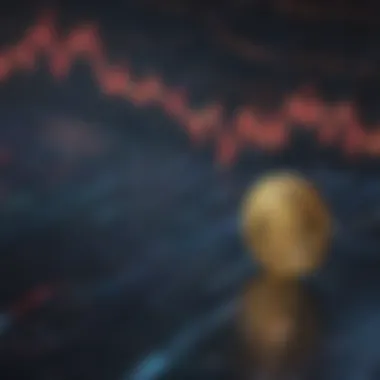
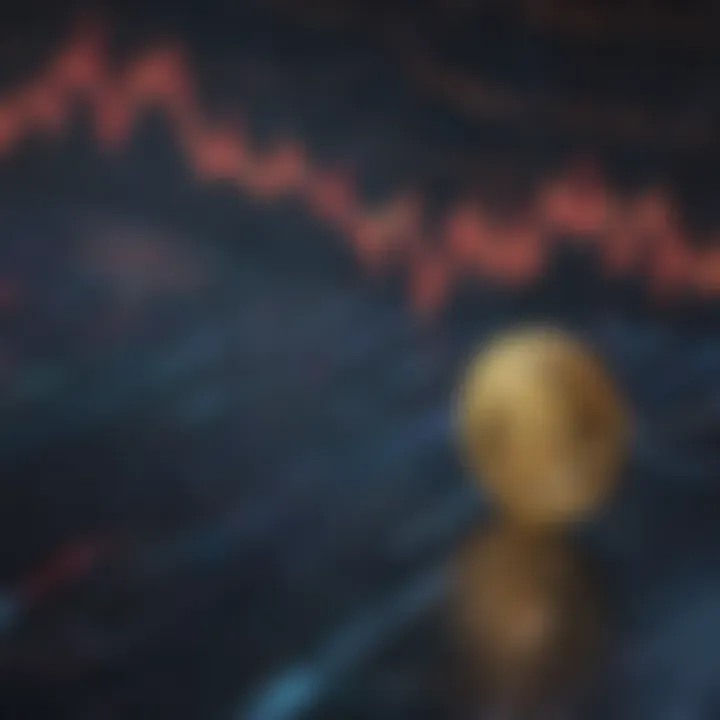
Intro
The world of trading has evolved dramatically over the past couple of decades, with quantitative trading emerging as a powerful force that reshapes how markets operate. Unlike traditional trading strategies that rely heavily on intuition and experience, quantitative trading leverages mathematical models and algorithmic processes to make trading decisions. This shift not only improves the speed and accuracy of trades but also opens the door to strategies that were previously unthinkable. As cryptocurrencies continue to gain prominence, investors and traders alike are keen to dive into this intricate realm, aiming to capitalize on the plethora of opportunities it presents.
In this narrative, we focus on the fundamentals of quantitative trading, aiming to delineate its foundational elements, methodologies, and implications, particularly within the context of the cryptocurrency market. With clear sections guiding the reader, we will traverse topics like algorithm design, risk management, and performance evaluation, allowing for a structured understanding of the system’s inner workings.
Furthermore, we will also explore how technology intertwines with market dynamics—a crucial aspect as we navigate an increasingly data-driven trading landscape. The insights gained can be invaluable for not just traders and investors but also for analysts and educators looking to comprehend the evolving nature of finance in light of digital advancements.
Market Trends and Analysis
Current Market Overview
The cryptocurrency market is a kaleidoscope of volatility and opportunity. Prices swing wildly, sometimes within the same day. Recent years have borne witness to rising trends, such as the dominance of Bitcoin, the emergence of Ethereum as a go-to for decentralized applications, and the burgeoning of various altcoins that cater to niche markets. Understanding these trends is pivotal for any quantitative trader looking to penetrate this space.
For instance, Bitcoin's surge to all-time highs can often coincide with global economic uncertainties, highlighting its perceived value as a digital gold. Conversely, Ethereum's developments in smart contracts have made it a favorite for many decentralized finance (DeFi) applications.
Some noteworthy aspects include:
- Market Capitalization: Tracking the rise and fall of cryptographic currencies can indicate broader trends in market sentiment.
- Liquidity Levels: Analyzing trading volumes helps in assessing the viability of executing large trades without affecting market prices.
Influential Market Factors
Identifying the factors that impact market trends is paramount for those engaged in quantitative trading. This includes:
- Regulatory Environment: Governments around the world are slowly drafting rules that could dramatically affect trading practices and market stability.
- Technological Developments: Innovations like layer-2 scaling solutions and updates to existing blockchain protocols can shift trading dynamics significantly.
- Market Sentiment: News cycles, social media discussions, and community engagements can cause unpredictable fluctuations in asset pricing.
In this context, quantitatively analyzing data sets derived from various sources allows traders to craft predictive models.
"In trading, knowledge is not just power; it's the key to survival."
Blockchain Technology Insights
Technical Innovations
The technical fabric of blockchain technology is intrinsically linked to the success of quantitative trading in cryptocurrencies. Key innovations such as smart contracts and automated decentralized exchanges (DEXs) enhance the efficiency of trades and eliminate intermediary risks. The adoption of Oracles, which provide real-world data to blockchains, further broadens the horizons for algorithmic trading by enabling more complex and responsive trading strategies.
Use Cases in Various Industries
While the financial sector has quickly embraced quantitative trading, other industries are not far behind.
- Supply Chain Management: Companies are leveraging blockchain for transparent tracking and fraud prevention.
- Healthcare: Secure patient data management is being revolutionized using decentralized technologies.
- Real Estate: Fractional ownership and transparent title records are reshaping conventional investment avenues.
These use cases highlight the versatile nature of blockchain technology and underscore how quantitative trading methodologies can be adapted or adopted across different sectors.
Prolusion to Quantitative Trading
In today’s digital age, quantitative trading stands out as a pivotal force in the financial landscape. With the proliferation of data and advances in technology, this method has transformed how individuals and institutions engage with markets. Understanding quantitative trading isn't just for the elite traders or academic researchers; it's essential for anyone venturing into investing, asset management, or even just keeping an eye on market trends. The underpinnings of this trading style hold the promise of clarity in complex market scenarios, leveraging data to take calculated risks.
One of the primary benefits of quantitative trading is its ability to remove emotional biases from trading decisions. Algorithms execute trades based on pre-defined criteria, which can significantly enhance consistency and reliability. The drive for systematic strategies can contribute to better risk management and overall profitability, as traders focus on data rather than gut feelings. With increasing competition, this method has become a necessity rather than just an option, allowing participants to keep pace with rapidly changing market conditions.
Definition and Purpose
Quantitative trading can be defined succinctly as the use of mathematical models and automated systems to make trading decisions. In essence, it relies on a steady flow of data—market prices, trading volumes, economic indicators, and more—to predict future movement or identify pricing inefficiencies. The purpose here is quite straightforward: maximizing returns while minimizing risks using statistical methods that analyze historical data.
Initially, this trading strategy honed its roots in large financial institutions, but recent democratization of technology has enabled individual traders to harness similar techniques at a fraction of the costs. For many, the goal is to leverage algorithms that can execute multiple trades in seconds, thus capitalizing on fleeting opportunities that a human trader might miss.
Historical Context
To fully grasp the significance of quantitative trading today, we must take a step back and look at its evolution. The lineage of this practice traces back to the late twentieth century, when early finance academicians began applying mathematical models to trading strategies. The introduction of computers into trading during the 1980s marked a significant turning point; tasks that once took hours could now be completed in mere seconds.
However, it wasn’t until the dot-com bubble burst in the late 1990s that quantitative strategies gained significant attention. Market volatility pushed traders to seek methods that could outperform traditional approaches. As technology improved and the availability of data exploded, confidence in quantitative methods grew.
In the 2000s, hedge funds such as Renaissance Technologies and Two Sigma paved the way, blending complex algorithms with robust data analysis to yield astonishing returns. Now, nearly two decades later, quantitative trading is not confined to institutional players but has spilled over into the realm of retail investors, with various platforms and tools readily available.
As we delve further into this article, one must appreciate this juxtaposition of historical foundations and modern advancements. The ongoing evolution of quantitative trading reflects a continuous adaptation to market conditions and technological innovations, further underscoring its relevance in an era dominated by data.
Key Concepts in Quantitative Trading
The realm of quantitative trading is a sophisticated landscape where numbers speak louder than words. Understanding the key concepts in this field isn't just an academic exercise; it's a practical necessity for anyone looking to dive deep into the intricacies of trading success. These concepts lay the groundwork for traders, investors, and analysts alike, illustrating how data can be transformed into actionable strategies that can yield solid cash flow if handled with diligence.
Data Analysis and Interpretation
At the heart of quantitative trading lies data analysis. It's akin to mining for gold in a riverbed; one must sift through tons of data to unearth valuable insights. Traders depend heavily on historical data, not just to grasp past market movements but to predict future behaviors. This analysis often involves using a series of mathematical and statistical tools.
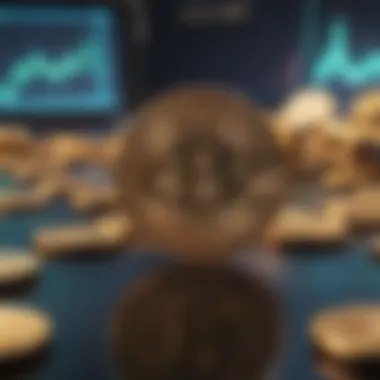
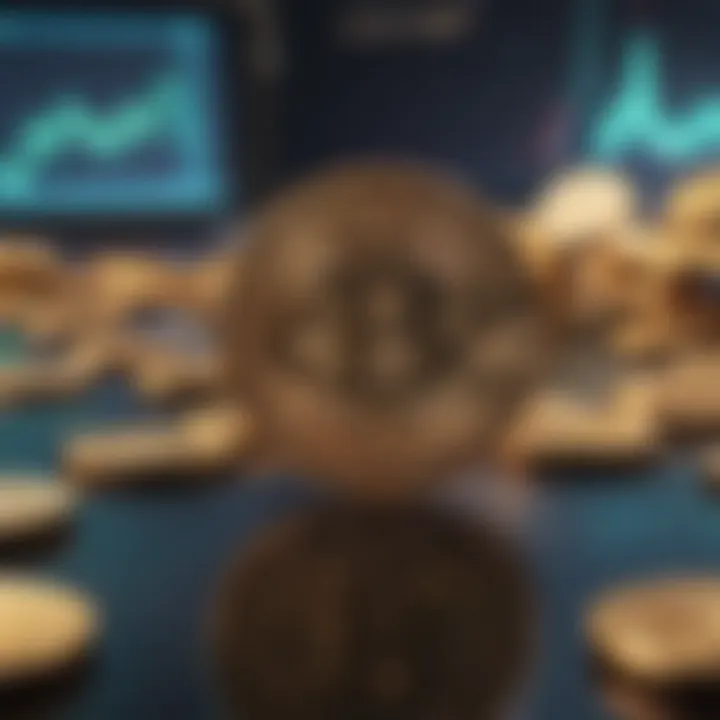
To effectively interpret data, one must consider various metrics, such as:
- Volatility - Measure how much the price of a security varies over time.
- Liquidity - Understand how easily an asset can be bought or sold without affecting its price.
- Correlation - Evaluate the relationship between multiple assets to identify potential trades.
There exists a crucial relationship between raw data and its interpretation. Misreading indicators might lead one to jump into a deal that could, in reality, be a slippery slope. Thus, a solid grounding in statistics is essential, as it allows traders to detect patterns that might otherwise evade the untrained eye.
Statistical Arbitrage
Statistical arbitrage is one of the pillars of quantitative trading strategies. Imagine you are at a supermarket where one item is offered at a reduced price, but you can sell it at a higher price at a different store. That’s the crux of statistical arbitrage. Traders exploit price discrepancies between related assets through mathematical models.
The approaches involved in statistical arbitrage include:
- A/B testing different trading strategies against historical data.
- Employing mean-reversion tactics where one assumes that prices will return to an average over time.
- Implementing pairs trading, which involves making simultaneous long and short trades on correlated securities.
However, it’s worth noting that market efficiency can pose a challenge here. As more players adopt similar strategies, opportunities quickly vanish. Thus, continual adaptation and rigorous backtesting are indispensable for remaining competitive.
Algorithmic Strategies
Algorithmic trading is like having a robotic assistant that works tirelessly to execute trades based on predefined criteria. These strategies allow traders to capitalize on minute market fluctuations that human traders may miss due to emotional biases or simply not being able to react quickly enough.
Key benefits of algorithmic strategies include:
- Speed - Algorithms can make trades in milliseconds, adjusting to market changes before a human could even blink.
- Elimination of Emotion - Trading decisions are based purely on data and analysis, reducing the risk of emotional-driven mistakes.
- Scalability - One developed algorithm can manage many trades across various markets or products concurrently.
Algorithms can be complex, integrating various models that consider multiple market factors. A common practice is to use machine learning algorithms, which continuously refine themselves as more data becomes available, enhancing decision-making capabilities over time.
Ultimately, to thrive in the landscape of quantitative trading, understanding these concepts is not just a non-negotiable; it’s essential. The familiarity with data analysis, statistical arbitrage, and algorithmic strategies transforms data into a robust toolkit. It's clear that mastery of these principles can set a solid foundation, empowering traders to navigate the fast-paced world of quantitative trading effectively.
The Role of Technology
In today’s financial landscape, technology stands as a cornerstone of quantitative trading. Its impact resonates through various aspects of trading, making it essential for any trader or investor seeking to navigate the complexities of the market. A robust grasp of technological tools and principles is indispensable. From data ingestion to execution of trading strategies, each step leverages technological advancements that result in enhanced performance.
Computational Efficiency
Computational efficiency is paramount in quantitative trading. The sheer volume of data produced in financial markets can overwhelm traditional analysis methods. Traders need swift processing power to analyze data and identify patterns. This demand has led to the rise of high-performance computing resources, which enable traders to run complex algorithms in real-time.
For example, the implementation of advanced CPUs and graphics processing units (GPUs) can significantly reduce the time needed for backtesting strategies. A trader can test years of data within minutes instead of days. This speed helps in identifying profitable strategies more effectively. Furthermore, computational efficiency allows for higher-frequency trading, where trades are executed in fractions of a second.
Software Tools and Platforms
Software tools and platforms are the lifeblood of quantitative trading. Traders rely on sophisticated software to build and deploy algorithmic trading strategies. Various platforms, like MetaTrader and NinjaTrader, offer traders granular control over their trading algorithms, allowing for customization to suit individual strategies.
- Backtesting Software: These tools allow traders to validate their algorithms against historical data, ensuring that their strategies have proven effective in past market conditions.
- Data Analysis Platforms: Tools such as R and Python’s Pandas library provide essential functions for data manipulation and visualization, enabling traders to draw insights swiftly.
Investors should consider the integration of cloud computing. This enables access to vast computational resources without the need for significant hardware investments. Being able to scale computing power allows traders to respond more adeptly to market changes, further proving the utility of technology in this space.
Machine Learning in Trading
Machine learning is adding an exciting layer to quantitative trading. With the ability to analyze massive datasets, machine learning algorithms can uncover patterns that humans might overlook. Traders are increasingly employing techniques like supervised and unsupervised learning for predictive analytics, enhancing the accuracy of their forecasts.
Here’s how machine learning fits into quantitative trading:
- Predicting Market Movements: Algorithms can learn from historical price data, sentiment analysis from news sources, or social media platforms to identify trends.
- Automated Trading Decisions: Once an algorithm identifies a potential opportunity, it can execute trades autonomously, reducing the lag associated with manual execution.
- Risk Assessment: Machine learning can also assist in improving risk management. It can analyze transaction patterns and alert traders about potential risks, enhancing decision-making processes.
By embracing machine learning, traders open themselves to a world of possibilities, forecasting market behavior and automating decisions with greater precision than ever before.
Understanding the role that technology plays in quantitative trading not only informs traders of the tools at their disposal but also highlights the importance of continual learning in this evolving landscape. As technology continues to advance, so too will the methods through which traders engage with the markets.
Market Dynamics and Trading Psychology
The landscape of quantitative trading is intricately woven with the threads of market dynamics and trading psychology. Understanding these elements is paramount for anyone who dabbles in this realm, as they shape not only market movements but also influence the decisions made by investors and traders. At its core, market dynamics deal with the behaviors of price movements, volumes, and liquidity. Conversely, trading psychology delves into the mental and emotional framework that underpins decision-making processes in the high-stakes world of trading.
Key aspects of market dynamics and trading psychology include:
- The way information is processed and acted upon in real-time.
- How trader emotions such as fear and greed can lead to irrational market behavior.
- The significance of understanding market trends, momentum, and reversals.
These considerations can provide a roadmap into the often unpredictable world of trading. A trader’s ability to navigate this landscape, applying quantitative techniques, can translate to successful trading strategies.
Behavioral Finance Considerations
Behavioral finance plays a critical role in overcoming traditional financial models that usually assume rationality among traders. This branch takes into account psychological factors that can lead individuals to deviate from rational decision-making. The interplay of cognitive biases and emotional reactions often distorts perceptions of risk and reward.
Consider these behavioral finance biases relevant to quantitative trading:
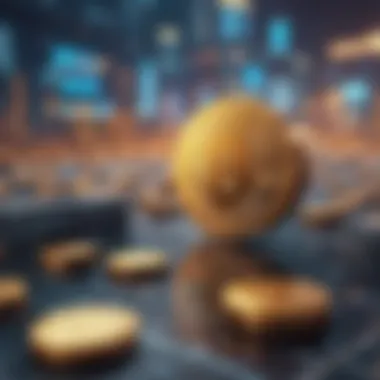
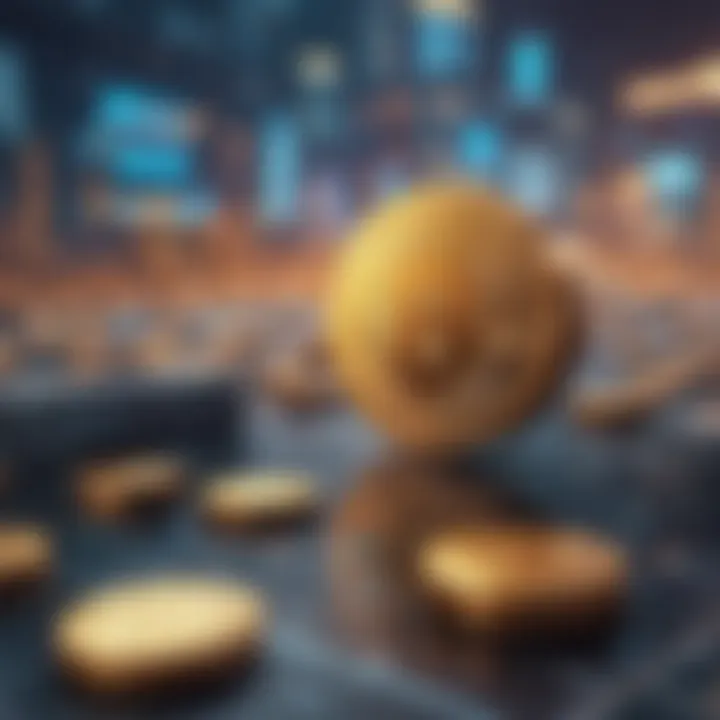
- Anchoring Bias: Traders may rely too heavily on the first piece of information they receive, such as past prices, which leads to poor decision-making.
- Herd Behavior: Individuals often follow the crowd, which can exacerbate market bubbles or crashes as people buy and sell en masse.
- Loss Aversion: Studies show that individuals prefer to avoid losses rather than acquiring equivalent gains, which alters their risk tolerance significantly.
Understanding these biases can help traders refine their algorithms and strategies to incorporate a more realistic view of market behavior. By anticipating how emotional responses can influence decisions during critical situations, traders can better prepare their strategies to maintain discipline.
Market Sentiment Analysis
Market sentiment analysis bridges the gap between quantitative techniques and psychological insights. It involves gauging the overall attitude of market participants toward specific securities or the market as a whole. Many traders use sentiment indicators derived from market data, news articles, and social media chatter to inform their decisions.
Some popular sentiment analysis techniques include:
- Sentiment Indicators: Tools like the Fear & Greed Index quantify market emotions based on factors like volatility and trading volume.
- News Sentiment Analysis: Evaluating the effects of press releases, articles, or social media activity can reveal trends that quantitative models alone might overlook.
- Investor Surveys: Gathering data directly from market participants can uncover sentiments that might not be reflected in price movements.
Engaging in sentiment analysis allows traders to complement their quantitative data with a qualitative understanding of market forces. By doing so, they can enhance their predictive models and bolster their market positioning, all while gaining crucial insight into the behavior of their competitors.
"In the world of trading, knowledge isn't just power; it's the difference between a calculated risk and a blind gamble."
In summary, a robust comprehension of market dynamics and trading psychology can lead to improved strategies, more effective risk management, and ultimately, better trading outcomes. The fusion of quantitative trading methods with behavioral finance insights allows investors to remain ahead of the curve in a constantly evolving marketplace.
Risk Management in Quantitative Trading
Risk management lies at the heart of quantitative trading, serving as the compass that guides traders through a landscape fraught with uncertainties. In a field driven by data and algorithms, understanding and mitigating risk is not just prudent; it's essential. Without proper risk management, even the most sophisticated algorithms can lead to catastrophic losses. This section unpacks the key components of risk management, emphasizing its critical role in the success of quantitative strategies.
Understanding Risk Metrics
To navigate the turbulent waters of trading, one must first grasp the essential metrics that define risk. Risk metrics are not merely numbers; they provide quantifiable insights into potential losses and market volatility. Here are some fundamental metrics used:
- Value at Risk (VaR): This metric estimates the potential loss in value of a position over a defined period for a given confidence interval. For instance, a VaR of $1 million at a 95% confidence level implies that there is a 5% chance of losing more than that amount over the specified period.
- Standard Deviation: A measure of the dispersion of returns. A high standard deviation indicates a higher risk, as prices can fluctuate significantly from their average values.
- Beta: This assesses the volatility of a stock or portfolio relative to the market. If a stock has a beta of 1.5, it’s expected to be 50% more volatile than the overall market.
Understanding these metrics allows traders to make informed decisions about which trades to pursue and how to adjust their portfolios to align with their risk appetite. It’s like having a clear map when embarking on a challenging hike.
Portfolio Diversification Tactics
Diversification acts as a safety valve for risk. By avoiding the pitfall of having all eggs in one basket, traders spread exposure across various instruments, asset classes, or markets. However, diversification in quantitative trading requires a strategic approach. Here are some tactics to consider:
- Asset Class Diversification: Involve different asset types like equities, bonds, commodities, or cryptocurrencies. This not only spreads risk but also capitalizes on different market cycles.
- Geographical Diversification: Investing in various regions can hedge against localized economic downturns. For instance, if a trader holds stocks in both the American and Asian markets, a downturn in one region might be offset by gains in another.
- Factor Diversification: Utilize different factors such as value, growth, momentum, or volatility. For instance, a portfolio that incorporates both growth stocks and value stocks can balance between aggressive and conservative investment strategies.
"Diversification is the only free lunch in investing."
The wisdom behind this saying underscores the effectiveness of diversification in managing risk while pursuing returns. As with cooking a flavorful dish, varying the ingredients often leads to a more satisfying outcome.
Stress Testing Models
Stress testing is a vital tool for understanding how a trading portfolio might react under extreme market conditions. Such models simulate different scenarios, often hypothetical, that could impact investments adversely. Here’s how traders can utilize them:
- Scenario Analysis: Traders create models based on historical crises and assess how their portfolio would have performed. For instance, analyzing the 2008 financial crisis can unveil vulnerabilities that might not be apparent during stable periods.
- Sensitivity Analysis: This technique examines how different variables impact outcomes. By adjusting one variable at a time, such as interest rates or market volatility, traders can see how sensitive their positions are to changes.
Stress testing not only uncovers weaknesses but also prompts adjustments to risk management strategies. If a particular model indicates significant potential losses in a bearish market, a trader might reconsider their positions or hedge their investments accordingly.
In summary, effective risk management in quantitative trading requires a multifaceted approach, incorporating understanding of risk metrics, diversification tactics, and stress testing. Each element serves to build a robust trading strategy that can weather market storms and capitalize on opportunities, enhancing overall investment success.
Performance Evaluation Techniques
Performance evaluation techniques are at the heart of quant trading, serving as a crucial lens through which traders scrutinize their strategies. Evaluating the effectiveness of trading algorithms is not just beneficial; it’s vital for long-term success in an ever-changing market landscape. Having a robust evaluation framework enables traders to understand the strengths and weaknesses of their strategies, guiding necessary adjustments and ultimately improving profitability.
Backtesting Methodologies
Backtesting is a method that allows traders to simulate their trading strategies using historical data. The process involves applying a trading strategy to past market conditions to assess how well it would have performed. This historical insight is invaluable because it provides a ground for validating a strategy before it is deployed in real-time trading.
Here are some key points to consider about backtesting:
- Data Quality: The accuracy and cleanliness of the historical data used for backtesting are essential. Using flawed data can lead to misleading results and poor decision-making.
- Market Conditions: Significant changes in market dynamics over time could render past performance irrelevant. For instance, strategies that thrived in a bull market may falter in a bearish environment.
- Overfitting: A common pitfall is creating a strategy that performs remarkably well on historical data but fails in the future due to overfitting. This occurs when the model is too complex and tailored to historical noise rather than genuine market patterns.
Backtesting methodologies help clarify the viability of strategies before risking capital. By examining various scenarios, traders gain insights into potential drawdowns and performance consistency.
Metrics for Measuring Success
Once a trading strategy has been backtested, the next step is the evaluation through metrics specifically designed to measure its success. These metrics provide quantitative assessments that enable comparisons between different strategies and help in refining them.
Some essential metrics include:
- Sharpe Ratio: It measures risk-adjusted return. A higher Sharpe ratio indicates that the strategy has delivered better returns per unit of risk.
- Maximum Drawdown: This is the largest observed loss from a peak to a trough during a specific period. Keeping this metric in check is critical, as high drawdowns can be detrimental to a trader's psychological and financial state.
- Win Rate: This metric reflects the percentage of trades that were profitable. While a high win rate is attractive, understanding the average payoff on winning trades compared to losing trades adds context.
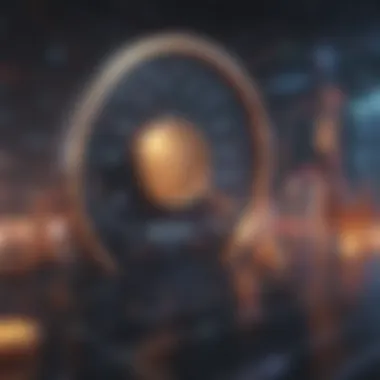
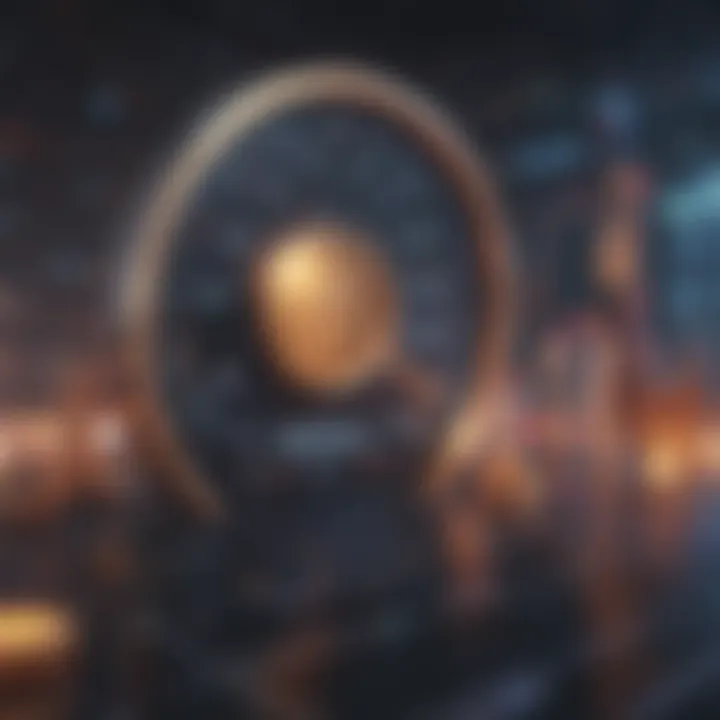
To strengthen your trading strategies, consider looking beyond metrics of success. Understanding how and when a strategy falters is as important as knowing when it thrives.
By utilizing these performance evaluation techniques, traders can not only validate their current strategies but also unveil areas for improvement. Ultimately, the goal is to build a resilient trading approach that can adapt over time and withstand market uncertainties.
Challenges and Limitations
In the realm of quantitative trading, challenges and limitations lurk like dark clouds, ready to rain on the parade of even the most seasoned traders. Addressing these matters is paramount, as they can fundamentally shape not only trading strategies but also the decisions that investors make. Understanding these barriers allows for a more grounded approach to trading, and often, success in this field hinges on navigating these hurdles deftly.
Data Quality Issues
When it comes to quantitative trading, the adage "garbage in, garbage out" rings particularly true. Data serves as the lifeblood of any trading model. If that data is tainted or faulty, the entire operation can turn sour quicker than you can say "market crash". Investors rely heavily on historical data to build models that predict future trends, but this data can be rife with inconsistencies.
- Sourcing Data: It isn't only about having an abundance of data, but more about having reliable sources. Public data from various exchanges can vary significantly, leading to discrepancies in analysis. Quantitative traders should often turn to established datasets and refrain from hasty assumptions based on fluctuating numbers.
- Real-time versus Historical Data: The difference between real-time data and historical data can lead to perilous decisions. If a trader bases their analysis on outdated information, the results can be misleading.
Consequently, ensuring that the data is not just large but also clean and relevant is one tough nut to crack in this trading landscape.
Market Adaptability
Markets are like chameleons; they adapt and change when least expected. The strategies that once were golden can quickly become outdated, showcasing the need for constant evolution. This adaptability can impede trading success.
- Changing Market Conditions: What works today might not work tomorrow. For instance, a model that performed spectacularly amidst a bull market can face challenges during a bear market. This volatility necessitates frequent alterations to existing strategies.
- Behavioral Dynamics: Markets are influenced by human emotions and irrational behaviors. Changes in global sentiment can make predictable patterns obsolete overnight.
Because of these shifting sands, traders must maintain a flexible mindset, ready to pivot strategies even on a dime. The trade-off? The longer it takes to adapt, the more profits can be eroded.
Regulatory Considerations
Let's face it, tread these waters poorly, and the regulatory sharks might just circle in for the kill. Quantitative trading isn't merely about numbers and algorithms; understanding the regulatory framework is crucial. Laws vary by geography and can change unexpectedly.
- Staying Compliant: Regulations such as the GDPR in Europe can have a significant impact on data usage. Failing to comply might lead to heavy penalties.
- Reporting Requirements: Different jurisdictions require various levels of transparency, demanding traders to remain vigilant and updated with local regulations.
As a result, staying ahead of the compliance curve can be both daunting and essential for long-term success. Ignorance of law is no excuse, as traders must constantly juggle the demands of effective trading with the need to follow the authorities' rules carefully.
In summation, while quantitative trading offers a miriad of opportunities, it is not without its challenges. From data quality issues to adapting to market ebb and flow, and complying with ever-changing regulations, each obstacle requires a strategic response to thrive in this dynamic environment.
The Future of Quantitative Trading
The future of quantitative trading holds immense significance in the ever-evolving landscape of financial markets. As trading methodologies continue to grow more sophisticated, a clear understanding of emerging trends, innovations, and the impact of technology on trading can empower investors and traders alike to make informed decisions. This section addresses several crucial aspects of this future, including new approaches to strategy development and performance evaluation, as well as the shifting role of traditional trading environments.
Emerging Trends and Innovations
The rapidly changing nature of financial markets drives the emergence of numerous trends and innovations in quantitative trading. Keeping an eye on these shifts offers traders a strategic advantage.
- Increased Use of Decentralized Finance (DeFi): The rise of decentralized finance has altered traditional trading paradigms. Smart contracts and decentralized exchanges offer new liquidity provisioning ideas, allowing traders to engage in more complex arbitrage opportunities.
- Cross-Asset Trading Strategies: Traders are increasingly looking beyond a single asset class. By combining various assets such as equities, cryptocurrencies, and commodities within a quantitative framework, traders can gain unique insights and potentially improve performance.
- Real-Time Data Utilization: The ability to process and analyze data in real-time is crucial. Traders are harnessing advancements in data infrastructure, enabling more effective integration of real-time market data into their quantitative models.
- Integration of Alternative Data: There's a growing trend to use alternative data sources such as satellite imagery, social media sentiment, or web scraping for quantitative analysis. These sources provide unique market insights not captured by traditional financial data, allowing traders to adjust their strategies accordingly.
Investors need to remain vigilant about these emerging trends to ensure their strategies are aligned with future market expectations.
The Role of AI and Big Data
Artificial intelligence and big data have become critical components of quantitative trading, shaping the methodologies that traders employ.
- Data-Driven Decisions: AI algorithms can analyze vast datasets at speeds humans cannot match, allowing for more informed and timely trading decisions. Traders can leverage machine learning techniques to identify patterns and correlations that were previously overlooked.
- Dynamic Strategy Adjustments: The adaptability of AI models enables them to adjust trading strategies based on real-time market conditions. This flexibility helps mitigate risks and capture new opportunities swiftly.
- Predictive Analytics: Utilizing advanced predictive analytics is no longer a luxury; it has become essential. Quantitative traders employ AI-assisted predictive models that forecast market trends based on historical data and various influencing factors.
- Automated Execution: The integration of AI aids in automating the execution of trades. Algorithmic trading platforms can independently execute trades based on predefined criteria, enhancing efficiency and reducing human error.
The synergy of AI and big data in quantitative trading not only improves trading performance but also contributes to a more holistic understanding of market dynamics.
"In the age of information overload, those who harness the power of data and AI will inevitably lead the market."
As quantitative traders prepare for the future, they must stay informed about these advancements, ensuring that they can leverage these tools to navigate an increasingly complex trading environment.
Finale
In concluding our exploration of quantitative trading, it becomes essential to emphasize the substantial relevance of this topic in today’s financial landscape. The evolution of trading strategies has increasingly gravitated towards algorithmic methods, primarily driven by the exponentially growing data availability and technological advancements. Understanding quantitative trading not only sheds light on how trades are executed efficiently but also reveals the intricacies that come along, such as risk management and performance evaluation.
Reflecting on the Evolution of Trading Strategies
Over the decades, trading strategies have transitioned from traditional methods driven largely by intuition and basic analysis to sophisticated systems relying on mathematical models and algorithms. This change can be attributed to various factors:
- Technological Advancements: Development in computational capabilities has allowed traders to analyze vast amounts of data in milliseconds. The transition from manual trading to automated systems has fundamentally altered the landscape, fostering quicker decision-making processes.
- Data Proliferation: The accessibility of high-quality market data has empowered quantitative trading. Investors who employ quantitative strategies can utilize historical data, statistical patterns, and predictive analytics to inform their trading decisions, resulting in more informed actions.
- Increased Market Efficiency: As quantitative strategies proliferate, the market becomes more efficient, meaning price discrepancies are identified and corrected quicker than before. This shift affects how traders strategize, leading to the development of more advanced techniques.
Reflecting on this evolution, it’s clear that the advent of quantitative trading enables traders to remain ahead of the curve. The ability to harness data effectively allows for better-informed strategies in a complex market.
Final Thoughts on the Quantitative Approach
- Improved Decision-Making: By relying on data and algorithms, investors can avoid emotional biases that often cloud judgment in market environments. Decisions are based on statistical evidence rather than mere speculation.
- Versatility Across Markets: This approach is not limited to traditional markets; it has been adaptable and finds use in diverse arenas, such as cryptocurrencies and forex trading.
- Scalability and Automation: Quantitative trading strategies can be scaled rapidly, which is crucial in today’s fast-paced financial environment. Automated trading algorithms can operate round-the-clock, capitalizing on market movements that human traders might miss.
Therefore, as we look forward into the realm of quantitative trading, the emphasis on continuous learning, adaptation, and effective risk management becomes paramount. Embracing these methodologies not only enhances individual trading practices but also contributes significantly to the broader market efficiency, offering a promising outlook for the future.
"The future of trading rests not just with the traders but with the technologies that empower and influence their strategies."
This structured understanding informs how individuals approach investing in this data-driven world. It opens the door for potential profits while underscoring the need for a strategic mindset in navigating today’s markets.